Lecture 8: Model transparency and conclusion#
UBC Master of Data Science program, 2022-23
Instructor: Varada Kolhatkar
Learning outcomes#
From this lecture, students are expected to be able to:
Explain why are transparency and interpretability important in ML.
Use
feature_importances_
attribute ofsklearn
models and interpret its output.Broadly explain how permutation feature importances are calculated and use
sklearn
’spermutation_importance
.Use
eli5
to get feature importances of nonsklearn
models and interpret its output.Create shapely values associated with tree-based models using the SHAP tool.
Generate and interpret the force plot to explain a specific prediction.
Explain summary and dependence plots produced with shapely values.
Learn to be aware of multicolinearity in your data when interpreting models.
Imports and LO#
Imports#
import os
import sys
sys.path.append("code/.")
import string
from collections import deque
import matplotlib.pyplot as plt
%matplotlib inline
import numpy as np
import pandas as pd
from plotting_functions import *
from sklearn import datasets
from sklearn.compose import ColumnTransformer, make_column_transformer
from sklearn.dummy import DummyClassifier
from sklearn.ensemble import RandomForestClassifier
from sklearn.impute import SimpleImputer
from sklearn.linear_model import LogisticRegression, Ridge, RidgeCV
from sklearn.model_selection import (
cross_validate,
train_test_split,
)
from sklearn.pipeline import Pipeline, make_pipeline
from sklearn.preprocessing import (
OneHotEncoder,
OrdinalEncoder,
PolynomialFeatures,
StandardScaler,
)
from sklearn.inspection import permutation_importance
from utils import *
Transparency and explainability of ML models: Motivation#
Activity (~5 mins)#
Suppose you have a machine learning model which gives you a 98% cross-validation score (with the metric of your interest) and 97% test score on a reasonably sized train and test sets. Since you have impressive cross-validation and test scores, you decide to just trust the model and use it as a black box, ignoring why it’s making certain predictions.
Give some scenarios when this might or might not be problematic. Write your thoughts in this Google document.
Why model transparency/interpretability?#
Ability to interpret ML models is crucial in many applications such as banking, healthcare, and criminal justice.
It can be leveraged by domain experts to diagnose systematic errors and underlying biases of complex ML systems.
What is model interpretability?#
In this lecture, our definition of model interpretability will be looking at feature importances, i.e., exploring features which are important to the model.
There is more to interpretability than feature importances, but it’s a good start!
Resources:
Data#
Let’s work with the adult census data set from the last lecture.
adult_df_large = pd.read_csv("data/adult.csv")
train_df, test_df = train_test_split(adult_df_large, test_size=0.2, random_state=42)
train_df_nan = train_df.replace("?", np.NaN)
test_df_nan = test_df.replace("?", np.NaN)
train_df_nan.head()
age | workclass | fnlwgt | education | education.num | marital.status | occupation | relationship | race | sex | capital.gain | capital.loss | hours.per.week | native.country | income | |
---|---|---|---|---|---|---|---|---|---|---|---|---|---|---|---|
5514 | 26 | Private | 256263 | HS-grad | 9 | Never-married | Craft-repair | Not-in-family | White | Male | 0 | 0 | 25 | United-States | <=50K |
19777 | 24 | Private | 170277 | HS-grad | 9 | Never-married | Other-service | Not-in-family | White | Female | 0 | 0 | 35 | United-States | <=50K |
10781 | 36 | Private | 75826 | Bachelors | 13 | Divorced | Adm-clerical | Unmarried | White | Female | 0 | 0 | 40 | United-States | <=50K |
32240 | 22 | State-gov | 24395 | Some-college | 10 | Married-civ-spouse | Adm-clerical | Wife | White | Female | 0 | 0 | 20 | United-States | <=50K |
9876 | 31 | Local-gov | 356689 | Bachelors | 13 | Married-civ-spouse | Prof-specialty | Husband | White | Male | 0 | 0 | 40 | United-States | <=50K |
numeric_features = ["age", "capital.gain", "capital.loss", "hours.per.week"]
categorical_features = [
"workclass",
"marital.status",
"occupation",
"relationship",
"native.country",
]
ordinal_features = ["education"]
binary_features = ["sex"]
drop_features = ["race", "education.num", "fnlwgt"]
target_column = "income"
education_levels = [
"Preschool",
"1st-4th",
"5th-6th",
"7th-8th",
"9th",
"10th",
"11th",
"12th",
"HS-grad",
"Prof-school",
"Assoc-voc",
"Assoc-acdm",
"Some-college",
"Bachelors",
"Masters",
"Doctorate",
]
assert set(education_levels) == set(train_df["education"].unique())
numeric_transformer = make_pipeline(SimpleImputer(strategy="median"), StandardScaler())
tree_numeric_transformer = make_pipeline(SimpleImputer(strategy="median"))
categorical_transformer = make_pipeline(
SimpleImputer(strategy="constant", fill_value="missing"),
OneHotEncoder(handle_unknown="ignore"),
)
ordinal_transformer = make_pipeline(
SimpleImputer(strategy="constant", fill_value="missing"),
OrdinalEncoder(categories=[education_levels], dtype=int),
)
binary_transformer = make_pipeline(
SimpleImputer(strategy="constant", fill_value="missing"),
OneHotEncoder(drop="if_binary", dtype=int),
)
preprocessor = make_column_transformer(
("drop", drop_features),
(numeric_transformer, numeric_features),
(ordinal_transformer, ordinal_features),
(binary_transformer, binary_features),
(categorical_transformer, categorical_features),
)
X_train = train_df_nan.drop(columns=[target_column])
y_train = train_df_nan[target_column]
X_test = test_df_nan.drop(columns=[target_column])
y_test = test_df_nan[target_column]
# encode categorical class values as integers for XGBoost
from sklearn.preprocessing import LabelEncoder
label_encoder = LabelEncoder()
y_train_num = label_encoder.fit_transform(y_train)
y_test_num = label_encoder.transform(y_test)
Do we have class imbalance?#
There is class imbalance. But without any context, both classes seem equally important.
Let’s use accuracy as our metric.
train_df_nan["income"].value_counts(normalize=True)
<=50K 0.757985
>50K 0.242015
Name: income, dtype: float64
scoring_metric = "accuracy"
Let’s store all the results in a dictionary called results
.
results = {}
We are going to use models outside sklearn. Some of them cannot handle categorical target values. So we’ll convert them to integers using LabelEncoder
.
y_train_num
array([0, 0, 0, ..., 1, 1, 0])
Baseline#
dummy = DummyClassifier()
results["Dummy"] = mean_std_cross_val_scores(
dummy, X_train, y_train_num, return_train_score=True, scoring=scoring_metric
)
Different models#
from lightgbm.sklearn import LGBMClassifier
from xgboost import XGBClassifier
pipe_lr = make_pipeline(
preprocessor, LogisticRegression(max_iter=2000, random_state=123)
)
pipe_rf = make_pipeline(preprocessor, RandomForestClassifier(random_state=123))
pipe_xgb = make_pipeline(
preprocessor, XGBClassifier(random_state=123, eval_metric="logloss", verbosity=0)
)
pipe_lgbm = make_pipeline(preprocessor, LGBMClassifier(random_state=123))
classifiers = {
"logistic regression": pipe_lr,
"random forest": pipe_rf,
"XGBoost": pipe_xgb,
"LightGBM": pipe_lgbm,
}
for (name, model) in classifiers.items():
results[name] = mean_std_cross_val_scores(
model, X_train, y_train_num, return_train_score=True, scoring=scoring_metric
)
pd.DataFrame(results).T
fit_time | score_time | test_score | train_score | |
---|---|---|---|---|
Dummy | 0.002 (+/- 0.001) | 0.000 (+/- 0.000) | 0.758 (+/- 0.000) | 0.758 (+/- 0.000) |
logistic regression | 0.477 (+/- 0.030) | 0.010 (+/- 0.002) | 0.849 (+/- 0.005) | 0.850 (+/- 0.001) |
random forest | 4.947 (+/- 0.149) | 0.087 (+/- 0.013) | 0.848 (+/- 0.006) | 0.979 (+/- 0.000) |
XGBoost | 0.837 (+/- 0.411) | 0.016 (+/- 0.004) | 0.870 (+/- 0.004) | 0.898 (+/- 0.001) |
LightGBM | 0.449 (+/- 0.076) | 0.017 (+/- 0.000) | 0.872 (+/- 0.004) | 0.888 (+/- 0.000) |
Logistic regression is giving reasonable scores but not the best ones.
XGBoost and LightGBM are giving us the best CV scores.
Often simple models (e.g., linear models) are interpretable but not very accurate.
Complex models (e.g., LightGBM) are more accurate but less interpretable.
Feature importances in linear models#
Let’s create and fit a pipeline with preprocessor and logistic regression.
pipe_lr = make_pipeline(preprocessor, LogisticRegression(max_iter=2000, random_state=2))
pipe_lr.fit(X_train, y_train_num);
ohe_feature_names = (
pipe_rf.named_steps["columntransformer"]
.named_transformers_["pipeline-4"]
.named_steps["onehotencoder"]
.get_feature_names_out(categorical_features)
.tolist()
)
feature_names = (
numeric_features + ordinal_features + binary_features + ohe_feature_names
)
feature_names[:15]
['age',
'capital.gain',
'capital.loss',
'hours.per.week',
'education',
'sex',
'workclass_Federal-gov',
'workclass_Local-gov',
'workclass_Never-worked',
'workclass_Private',
'workclass_Self-emp-inc',
'workclass_Self-emp-not-inc',
'workclass_State-gov',
'workclass_Without-pay',
'workclass_missing']
data = {
"coefficient": pipe_lr.named_steps["logisticregression"].coef_.flatten().tolist(),
"magnitude": np.absolute(
pipe_lr.named_steps["logisticregression"].coef_.flatten().tolist()
),
}
coef_df = pd.DataFrame(data, index=feature_names).sort_values(
"magnitude", ascending=False
)
coef_df[:10]
coefficient | magnitude | |
---|---|---|
capital.gain | 2.356032 | 2.356032 |
marital.status_Married-AF-spouse | 1.761385 | 1.761385 |
occupation_Priv-house-serv | -1.426083 | 1.426083 |
marital.status_Married-civ-spouse | 1.347748 | 1.347748 |
relationship_Wife | 1.289777 | 1.289777 |
native.country_Columbia | -1.080529 | 1.080529 |
occupation_Prof-specialty | 1.078725 | 1.078725 |
occupation_Exec-managerial | 1.056150 | 1.056150 |
native.country_Dominican-Republic | -1.009499 | 1.009499 |
relationship_Own-child | -0.985885 | 0.985885 |
Increasing
capital.gain
is likely to push the prediction towards “>50k” income classWhereas occupation of private house service is likely to push the prediction towards “<=50K” income.
Can we get feature importances for non-linear models?
Model interpretability beyond linear models#
We will be looking at interpretability in terms of feature importances.
Note that there is no absolute or perfect way to get feature importances. But it’s useful to get some idea on feature importances. So we just try our best.
We will be looking at three ways to get feature importances.
sklearn
’sfeature_importances_
andpermutation_importance
eli5 (stands for “explain like I’m 5”)
sklearn
’s feature_importances_
and permutation_importance
#
Feature importance or variable importance is a score associated with a feature which tells us how “important” the feature is to the model.
Activity (~5 mins)#
Linear models learn a coefficient associated with each feature which tells us the importance of the feature to the model.
What might be some reasonable ways to calculate feature importances of the following models?
Decision trees
Linear SVMs
KNNs, RBF SVMs
Suppose you have correlated features in your dataset. Do you need to be careful about this when you examine feature importances?
Discuss with your neighbour and write your ideas in this Google doc.
sklearn
’s feature_importances_
attribute vs permutation_importance
#
Feature importances can be
algorithm dependent, i.e., calculated based on the information given by the model algorithm (e.g., gini importance)
model agnostic (e.g., by measuring increase in prediction error after permuting feature values).
Different measures give insight into different aspects of your data and model.
Here you will find some drawbacks of using
feature_importances_
attribute in the context of tree-based models.
Decision tree feature importances#
pipe_dt = make_pipeline(preprocessor, DecisionTreeClassifier(max_depth=3))
pipe_dt.fit(X_train, y_train_num);
data = {
"Importance": pipe_dt.named_steps["decisiontreeclassifier"].feature_importances_,
}
pd.DataFrame(data=data, index=feature_names,).sort_values(
by="Importance", ascending=False
)[:10]
Importance | |
---|---|
marital.status_Married-civ-spouse | 0.543351 |
capital.gain | 0.294855 |
education | 0.160727 |
age | 0.001068 |
native.country_Guatemala | 0.000000 |
native.country_Iran | 0.000000 |
native.country_India | 0.000000 |
native.country_Hungary | 0.000000 |
native.country_Hong | 0.000000 |
native.country_Honduras | 0.000000 |
display_tree(feature_names, pipe_dt.named_steps["decisiontreeclassifier"], counts=True)
Let’s explore permutation importance.
For each feature this method evaluates the impact of permuting feature values
def get_permutation_importance(model):
X_train_perm = X_train.drop(columns=["race", "education.num", "fnlwgt"])
result = permutation_importance(model, X_train_perm, y_train_num, n_repeats=10, random_state=123)
perm_sorted_idx = result.importances_mean.argsort()
plt.boxplot(
result.importances[perm_sorted_idx].T,
vert=False,
labels=X_train_perm.columns[perm_sorted_idx],
)
plt.xlabel('Permutation feature importance')
plt.show()
get_permutation_importance(pipe_dt)
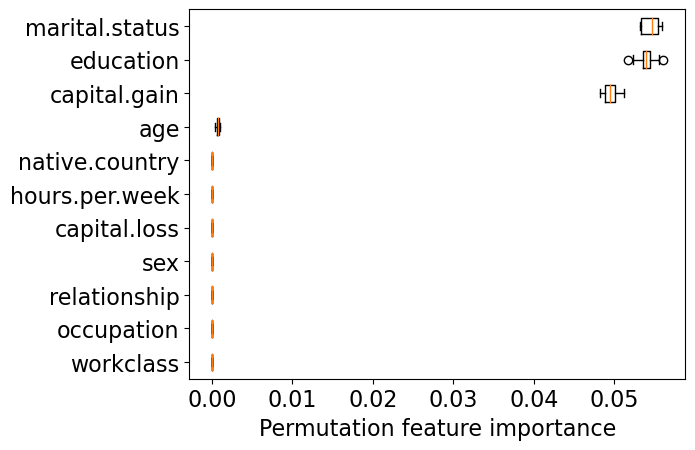
Decision tree is primarily making all decisions based on three features: marital.status, education, and capital.gain.
Let’s create and fit a pipeline with preprocessor and random forest.
Random forest feature importances#
pipe_rf = make_pipeline(preprocessor, RandomForestClassifier(random_state=2))
pipe_rf.fit(X_train, y_train_num);
Which features are driving the predictions the most?
data = {
"Importance": pipe_rf.named_steps["randomforestclassifier"].feature_importances_,
}
rf_imp_df = pd.DataFrame(
data=data,
index=feature_names,
).sort_values(by="Importance", ascending=False)
rf_imp_df[:8]
Importance | |
---|---|
age | 0.229718 |
education | 0.121846 |
hours.per.week | 0.114966 |
capital.gain | 0.114766 |
marital.status_Married-civ-spouse | 0.077204 |
relationship_Husband | 0.044253 |
capital.loss | 0.038707 |
marital.status_Never-married | 0.025489 |
np.sum(pipe_rf.named_steps["randomforestclassifier"].feature_importances_)
1.0
get_permutation_importance(pipe_rf)
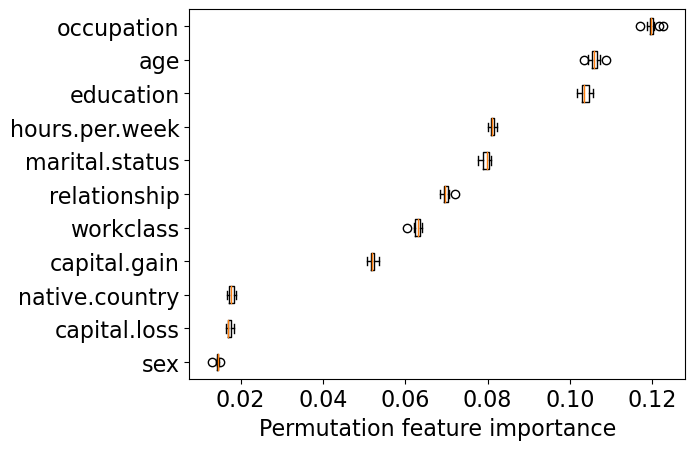
Random forest is using more features in the model compared to decision trees.
Key point#
Unlike the linear model coefficients,
feature_importances_
do not have a sign!They tell us about importance, but not an “up or down”.
Indeed, increasing a feature may cause the prediction to first go up, and then go down.
This cannot happen in linear models, because they are linear.
How can we get feature importances for non sklearn
models?#
One way to do it is by using a tool called
eli5
.
I don’t recall whether I included in the course conda environment or not. If not, you’ll have to install it:
conda install -c conda-forge eli5
Let’s look at feature importances for XGBClassifier
.
Note
For some reason, eli5.explain_weights
doesn’t work with the latest version of xgboost
. I downgraded the xgboost
version using this command to make it work conda install -c conda-forge py-xgboost==1.3.0
.
import eli5
pipe_xgb = make_pipeline(
preprocessor, XGBClassifier(random_state=123, eval_metric="logloss", verbosity=0)
)
pipe_xgb.fit(X_train, y_train_num)
eli5.explain_weights(pipe_xgb.named_steps["xgbclassifier"], feature_names=feature_names)
Weight | Feature |
---|---|
0.4838 | marital.status_Married-civ-spouse |
0.0477 | capital.gain |
0.0432 | occupation_Other-service |
0.0397 | relationship_Own-child |
0.0264 | education |
0.0243 | capital.loss |
0.0174 | occupation_Exec-managerial |
0.0167 | occupation_Prof-specialty |
0.0143 | occupation_Tech-support |
0.0138 | occupation_Handlers-cleaners |
0.0126 | occupation_Farming-fishing |
0.0121 | occupation_Machine-op-inspct |
0.0107 | workclass_Federal-gov |
0.0095 | age |
0.0086 | sex |
0.0085 | workclass_Self-emp-inc |
0.0085 | relationship_Wife |
0.0081 | relationship_Other-relative |
0.0080 | hours.per.week |
0.0075 | native.country_Philippines |
… 65 more … |
get_permutation_importance(pipe_xgb)
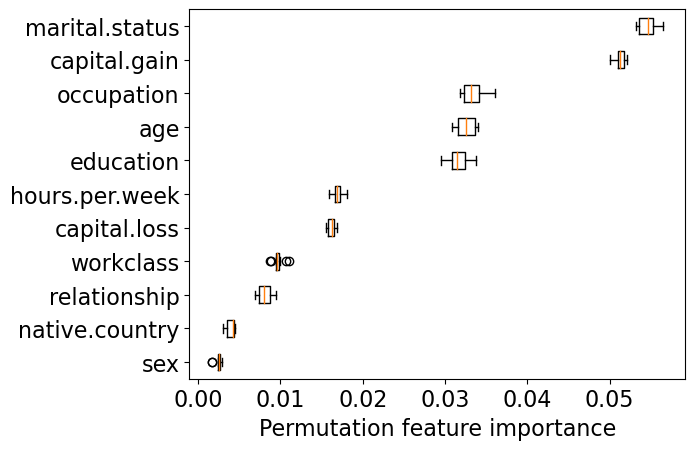
Let’s look at feature importances for LGBMClassifier
.
pipe_lgbm = make_pipeline(preprocessor, LGBMClassifier(random_state=123))
pipe_lgbm.fit(X_train, y_train_num)
eli5.explain_weights(
pipe_lgbm.named_steps["lgbmclassifier"], feature_names=feature_names
)
Weight | Feature |
---|---|
0.3613 | marital.status_Married-civ-spouse |
0.1941 | capital.gain |
0.1411 | education |
0.0890 | age |
0.0659 | capital.loss |
0.0440 | hours.per.week |
0.0131 | occupation_Exec-managerial |
0.0115 | occupation_Prof-specialty |
0.0072 | occupation_Other-service |
0.0063 | sex |
0.0056 | relationship_Wife |
0.0055 | workclass_Self-emp-not-inc |
0.0055 | occupation_Farming-fishing |
0.0054 | relationship_Own-child |
0.0030 | occupation_Tech-support |
0.0030 | occupation_Sales |
0.0026 | workclass_Federal-gov |
0.0026 | workclass_Private |
0.0023 | occupation_Handlers-cleaners |
0.0022 | workclass_Self-emp-inc |
… 65 more … |
marital.status_Married-civ-spouse
is showing up as an important feature in multiple models. Recall that this feature is highly correlated to relationship_Husband
, which is not showing up as an important feature. If we remove the former, the latter is likely to show up as an important feature.
corr_df['marital.status_Married-civ-spouse']['relationship_Husband']
0.8937442459553657
get_permutation_importance(pipe_lgbm)
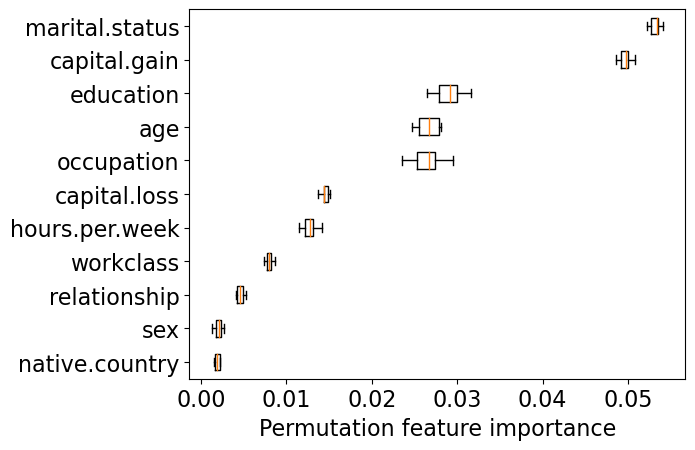
You can also look at feature importances for RandomForestClassifier
.
pipe_rf = make_pipeline(preprocessor, RandomForestClassifier(random_state=123))
pipe_rf.fit(X_train, y_train)
eli5.explain_weights(
pipe_rf.named_steps["randomforestclassifier"], feature_names=feature_names
)
Weight | Feature |
---|---|
0.2319 ± 0.0434 | age |
0.1172 ± 0.0309 | education |
0.1160 ± 0.0214 | hours.per.week |
0.1126 ± 0.0479 | capital.gain |
0.0694 ± 0.1408 | marital.status_Married-civ-spouse |
0.0474 ± 0.1132 | relationship_Husband |
0.0386 ± 0.0140 | capital.loss |
0.0271 ± 0.0747 | marital.status_Never-married |
0.0245 ± 0.0269 | occupation_Exec-managerial |
0.0221 ± 0.0201 | occupation_Prof-specialty |
0.0131 ± 0.0240 | sex |
0.0122 ± 0.0306 | relationship_Not-in-family |
0.0099 ± 0.0040 | workclass_Private |
0.0093 ± 0.0327 | relationship_Own-child |
0.0087 ± 0.0221 | marital.status_Divorced |
0.0086 ± 0.0180 | relationship_Wife |
0.0084 ± 0.0037 | workclass_Self-emp-not-inc |
0.0083 ± 0.0103 | occupation_Other-service |
0.0073 ± 0.0175 | relationship_Unmarried |
0.0071 ± 0.0061 | workclass_Self-emp-inc |
… 65 more … |
Let’s compare them with weights what we got with sklearn
feature_importances_
data = {
"Importance": pipe_rf.named_steps["randomforestclassifier"].feature_importances_,
}
pd.DataFrame(data=data, index=feature_names,).sort_values(
by="Importance", ascending=False
)[:10]
Importance | |
---|---|
age | 0.231860 |
education | 0.117203 |
hours.per.week | 0.116005 |
capital.gain | 0.112640 |
marital.status_Married-civ-spouse | 0.069385 |
relationship_Husband | 0.047355 |
capital.loss | 0.038566 |
marital.status_Never-married | 0.027144 |
occupation_Exec-managerial | 0.024527 |
occupation_Prof-specialty | 0.022069 |
These values tell us globally about which features are important.
But what if you want to explain a specific prediction.
Some fancier tools can help us do this.
Break#
SHAP (SHapley Additive exPlanations) introduction#
Explaining a prediction#
SHAP (SHapley Additive exPlanations)#
Based on the idea of shapely values. A shapely value is created for each example and each feature.
Can explain the prediction of an example by computing the contribution of each feature to the prediction.
Great visualizations
Support for different kinds of models; fast variants for tree-based models
Original paper: Lundberg and Lee, 2017
Our focus#
How to use it on our dataset?
How to generate and interpret plots created by SHAP?
We are not going to discuss how SHAP works.
Start at a base rate (e.g., how often people get their loans rejected).
Add one feature at a time and see how it impacts the decision.
SHAP on LGBM model#
Let’s try it out on our best performing LightGBM model.
You should have
shap
in the course conda environment
Let’s create train and test dataframes with our transformed features.
X_train_enc = pd.DataFrame(
data=preprocessor.transform(X_train).toarray(),
columns=feature_names,
index=X_train.index,
)
X_train_enc.head()
age | capital.gain | capital.loss | hours.per.week | education | sex | workclass_Federal-gov | workclass_Local-gov | workclass_Never-worked | workclass_Private | ... | native.country_Puerto-Rico | native.country_Scotland | native.country_South | native.country_Taiwan | native.country_Thailand | native.country_Trinadad&Tobago | native.country_United-States | native.country_Vietnam | native.country_Yugoslavia | native.country_missing | |
---|---|---|---|---|---|---|---|---|---|---|---|---|---|---|---|---|---|---|---|---|---|
5514 | -0.921955 | -0.147166 | -0.21768 | -1.258387 | 8.0 | 1.0 | 0.0 | 0.0 | 0.0 | 1.0 | ... | 0.0 | 0.0 | 0.0 | 0.0 | 0.0 | 0.0 | 1.0 | 0.0 | 0.0 | 0.0 |
19777 | -1.069150 | -0.147166 | -0.21768 | -0.447517 | 8.0 | 0.0 | 0.0 | 0.0 | 0.0 | 1.0 | ... | 0.0 | 0.0 | 0.0 | 0.0 | 0.0 | 0.0 | 1.0 | 0.0 | 0.0 | 0.0 |
10781 | -0.185975 | -0.147166 | -0.21768 | -0.042081 | 13.0 | 0.0 | 0.0 | 0.0 | 0.0 | 1.0 | ... | 0.0 | 0.0 | 0.0 | 0.0 | 0.0 | 0.0 | 1.0 | 0.0 | 0.0 | 0.0 |
32240 | -1.216346 | -0.147166 | -0.21768 | -1.663822 | 12.0 | 0.0 | 0.0 | 0.0 | 0.0 | 0.0 | ... | 0.0 | 0.0 | 0.0 | 0.0 | 0.0 | 0.0 | 1.0 | 0.0 | 0.0 | 0.0 |
9876 | -0.553965 | -0.147166 | -0.21768 | -0.042081 | 13.0 | 1.0 | 0.0 | 1.0 | 0.0 | 0.0 | ... | 0.0 | 0.0 | 0.0 | 0.0 | 0.0 | 0.0 | 1.0 | 0.0 | 0.0 | 0.0 |
5 rows × 85 columns
X_test_enc = pd.DataFrame(
data=preprocessor.transform(X_test).toarray(),
columns=feature_names,
index=X_test.index,
)
X_test_enc.shape
(6513, 85)
Let’s get SHAP values for train and test data.
import shap
# Create a shap explainer object
lgbm_explainer = shap.TreeExplainer(pipe_lgbm.named_steps["lgbmclassifier"])
train_lgbm_shap_values = lgbm_explainer.shap_values(X_train_enc)
LightGBM binary classifier with TreeExplainer shap values output has changed to a list of ndarray
train_lgbm_shap_values
[array([[ 4.08151507e-01, 2.82025568e-01, 4.70162085e-02, ...,
-1.03017665e-03, 0.00000000e+00, -1.69027185e-03],
[ 5.46019608e-01, 2.77536150e-01, 4.69698010e-02, ...,
-9.00720988e-04, 0.00000000e+00, -6.78058051e-04],
[-4.39095422e-01, 2.50475372e-01, 6.51137414e-02, ...,
-9.02446630e-04, 0.00000000e+00, -3.54676006e-04],
...,
[-1.05137470e+00, 1.89706451e-01, -2.74798624e+00, ...,
-1.13229595e-03, 0.00000000e+00, -1.31449687e-04],
[-6.32247597e-01, 3.01432486e-01, 8.99744241e-02, ...,
-1.03411038e-03, 0.00000000e+00, 4.04709519e-04],
[ 1.15559528e+00, 2.32397724e-01, 5.55862988e-02, ...,
-1.05290827e-03, 0.00000000e+00, -8.11336331e-04]]),
array([[-4.08151507e-01, -2.82025568e-01, -4.70162085e-02, ...,
1.03017665e-03, 0.00000000e+00, 1.69027185e-03],
[-5.46019608e-01, -2.77536150e-01, -4.69698010e-02, ...,
9.00720988e-04, 0.00000000e+00, 6.78058051e-04],
[ 4.39095422e-01, -2.50475372e-01, -6.51137414e-02, ...,
9.02446630e-04, 0.00000000e+00, 3.54676006e-04],
...,
[ 1.05137470e+00, -1.89706451e-01, 2.74798624e+00, ...,
1.13229595e-03, 0.00000000e+00, 1.31449687e-04],
[ 6.32247597e-01, -3.01432486e-01, -8.99744241e-02, ...,
1.03411038e-03, 0.00000000e+00, -4.04709519e-04],
[-1.15559528e+00, -2.32397724e-01, -5.55862988e-02, ...,
1.05290827e-03, 0.00000000e+00, 8.11336331e-04]])]
For each example, each feature, and each class we have a SHAP value.
SHAP values tell us how to fairly distribute the prediction among features.
For classification it’s a bit confusing. It gives SHAP matrix for all classes.
Let’s stick to shap values for class 1, i.e., income > 50K.
train_lgbm_shap_values[1].shape
(26048, 85)
test_lgbm_shap_values = lgbm_explainer.shap_values(X_test_enc)
test_lgbm_shap_values[1].shape
(6513, 85)
SHAP plots#
# load JS visualization code to notebook
shap.initjs()
Force plots#
Most useful!
Let’s try to explain predictions on a couple of examples from the test data.
I’m sampling some examples where target is <=50K and some examples where target is >50K.
y_test_reset = y_test.reset_index(drop=True)
y_test_reset
0 <=50K
1 <=50K
2 <=50K
3 <=50K
4 <=50K
...
6508 <=50K
6509 <=50K
6510 >50K
6511 <=50K
6512 >50K
Name: income, Length: 6513, dtype: object
l50k_ind = y_test_reset[y_test_reset == "<=50K"].index.tolist()
g50k_ind = y_test_reset[y_test_reset == ">50K"].index.tolist()
ex_l50k_index = l50k_ind[10]
ex_g50k_index = g50k_ind[10]
Explaining a prediction#
Imagine that you are given the following test example.
X_test_enc.iloc[ex_l50k_index]
age 0.476406
capital.gain -0.147166
capital.loss 4.649658
hours.per.week -0.042081
education 8.000000
...
native.country_Trinadad&Tobago 0.000000
native.country_United-States 1.000000
native.country_Vietnam 0.000000
native.country_Yugoslavia 0.000000
native.country_missing 0.000000
Name: 345, Length: 85, dtype: float64
You get the following hard prediction, which you are interested in explaining.
pipe_lgbm.named_steps["lgbmclassifier"].predict(X_test_enc)[ex_l50k_index]
0
You can first look at predict_proba
output to get a better understanding of model confidence.
pipe_lgbm.named_steps["lgbmclassifier"].predict_proba(X_test_enc)[ex_l50k_index]
array([0.99240562, 0.00759438])
The model seems quite confident. But if we want to know more, for example, which feature values are playing a role in this specific prediction, we can use SHAP force plots.
Remember that we have SHAP values per feature per example. We’ll use these values to create SHAP force plot.
pd.DataFrame(
test_lgbm_shap_values[1][ex_l50k_index, :],
index=feature_names,
columns=["SHAP values"],
)
SHAP values | |
---|---|
age | 0.723502 |
capital.gain | -0.253426 |
capital.loss | -0.256666 |
hours.per.week | -0.096692 |
education | -0.403715 |
... | ... |
native.country_Trinadad&Tobago | 0.000000 |
native.country_United-States | 0.003408 |
native.country_Vietnam | 0.001051 |
native.country_Yugoslavia | 0.000000 |
native.country_missing | 0.000663 |
85 rows × 1 columns
SHAP will produce the following type of plots.
shap.force_plot(
lgbm_explainer.expected_value[1], # expected value for class 1.
test_lgbm_shap_values[1][ex_l50k_index, :], # SHAP values associated with the example we want to explain
X_test_enc.iloc[ex_l50k_index, :], # Feature vector of the example
matplotlib=True,
)
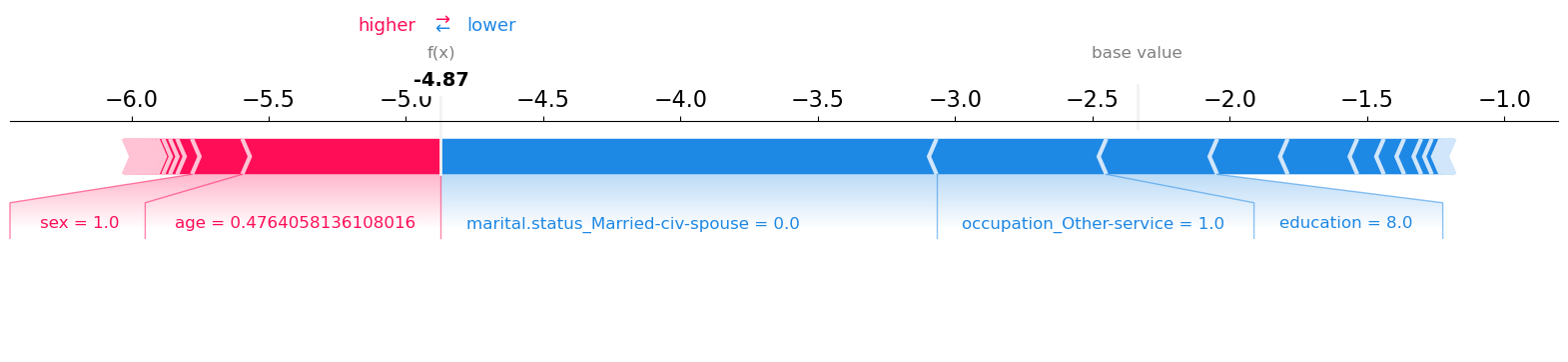
The raw model score is much smaller than the base value, which is reflected in the prediction of <= 50k class.
sex = 1.0, scaled age = 0.48 are pushing the prediction towards higher score.
education = 8.0, occupation_Other-service = 1.0 and marital.status_Married-civ-spouse = 0.0 are pushing the prediction towards lower score.
pipe_lgbm.named_steps["lgbmclassifier"].classes_
array([0, 1])
We can get the raw model output by passing raw_score=True
in predict
.
pipe_lgbm.named_steps["lgbmclassifier"].predict(X_test_enc, raw_score=True)
array([-1.76270194, -7.61912405, -0.45555535, ..., 1.13521135,
-6.62873917, -0.84062193])
What’s the raw score of the example above we are trying to explain?
pipe_lgbm.named_steps["lgbmclassifier"].predict(X_test_enc, raw_score=True)[ex_l50k_index]
-4.872722908439952
The score matches with what we see in the force plot.
The base score above is the mean raw score. Our example has a lower raw score compared to the average raw score and the force plot tries to explain which feature values are bringing this score to a lower value.
pipe_lgbm.named_steps["lgbmclassifier"].predict(X_train_enc, raw_score=True).mean()
-2.336411423367732
lgbm_explainer.expected_value[1] # on average this is the raw score for class 1
-2.3364114233677307
Note: a nice thing about SHAP values is that the feature importances sum to the prediction:
test_lgbm_shap_values[1][ex_l50k_index, :].sum() + lgbm_explainer.expected_value[1]
-4.8727229084399575
Now let’s try to explain another prediction.
The hard prediction here is 1.
From the
predict_proba
output it seems like the model is not too confident about the prediction.
pipe_lgbm.named_steps["lgbmclassifier"].predict(X_test_enc)[ex_g50k_index]
1
# X_test_enc.iloc[ex_g50k_index]
pipe_lgbm.named_steps["lgbmclassifier"].predict_proba(X_test_enc)[ex_g50k_index]
array([0.35997929, 0.64002071])
What’s the raw score for this example?
pipe_lgbm.named_steps["lgbmclassifier"].predict(X_test_enc, raw_score=True)[
ex_g50k_index
] # raw model score
0.5754540510801829
# pd.DataFrame(
# test_lgbm_shap_values[1][ex_g50k_index, :],
# index=feature_names,
# columns=["SHAP values"],
# )
shap.force_plot(
lgbm_explainer.expected_value[1],
test_lgbm_shap_values[1][ex_g50k_index, :],
X_test_enc.iloc[ex_g50k_index, :],
matplotlib=True,
)
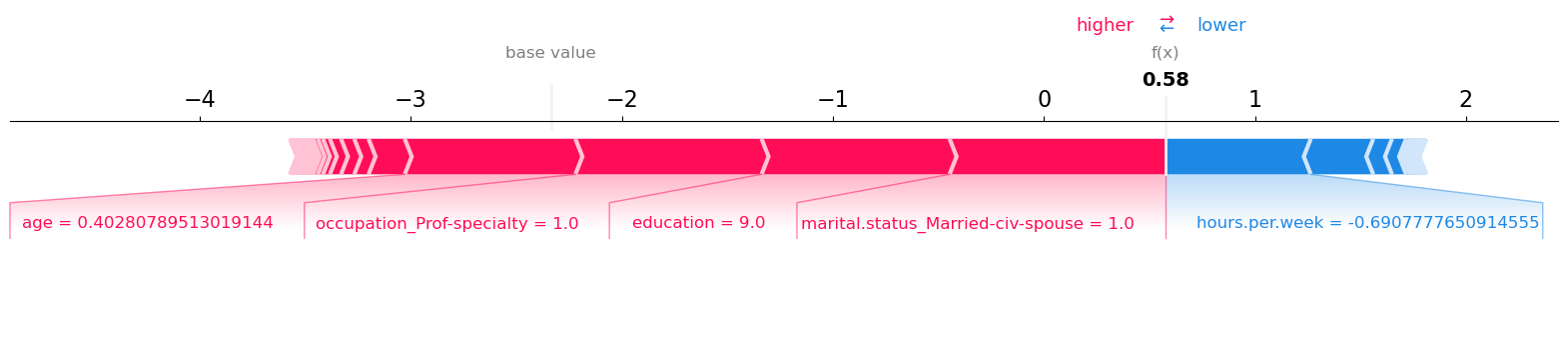
Observations:
Everything is with respect to class 1 here.
The base value, i.e., the average raw score for class 1 is -2.336.
We see the forces that drive the prediction.
That is, we can see the main factors pushing it from the base value (average over the dataset) to this particular prediction.
Features that push the prediction to a higher value are shown in red.
Features that push the prediction to a lower value are shown in blue.
Global feature importance using SHAP#
Let’s look at the average SHAP values associated with each feature.
values = np.abs(train_lgbm_shap_values[1]).mean(
0
) # mean of shapely values in each column
pd.DataFrame(data=values, index=feature_names, columns=["SHAP"]).sort_values(
by="SHAP", ascending=False
)[:10]
SHAP | |
---|---|
marital.status_Married-civ-spouse | 1.074859 |
age | 0.805468 |
capital.gain | 0.565589 |
education | 0.417642 |
hours.per.week | 0.324636 |
sex | 0.185687 |
capital.loss | 0.148519 |
marital.status_Never-married | 0.139914 |
relationship_Own-child | 0.108003 |
occupation_Prof-specialty | 0.106276 |
Dependence plot#
shap.dependence_plot("age", train_lgbm_shap_values[1], X_train_enc)
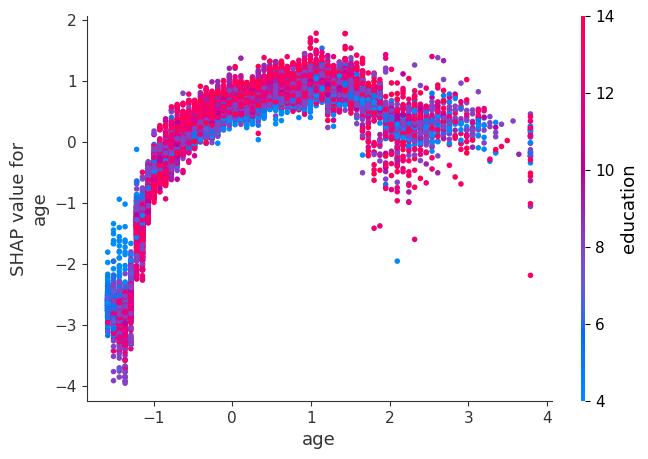
The plot above shows effect of age
feature on the prediction.
Each dot is a single prediction for examples above.
The x-axis represents values of the feature age (scaled).
The y-axis is the SHAP value for that feature, which represents how much knowing that feature’s value changes the output of the model for that example’s prediction.
Lower values of age have smaller SHAP values for class “>50K”.
Similarly, higher values of age also have a bit smaller SHAP values for class “>50K”, which makes sense.
There is some optimal value of age between scaled age of 1 which gives highest SHAP values for for class “>50K”.
Ignore the colour for now. The color corresponds to a second feature (education feature in this case) that may have an interaction effect with the feature we are plotting.
Summary plot#
shap.summary_plot(train_lgbm_shap_values[1], X_train_enc)
No data for colormapping provided via 'c'. Parameters 'vmin', 'vmax' will be ignored
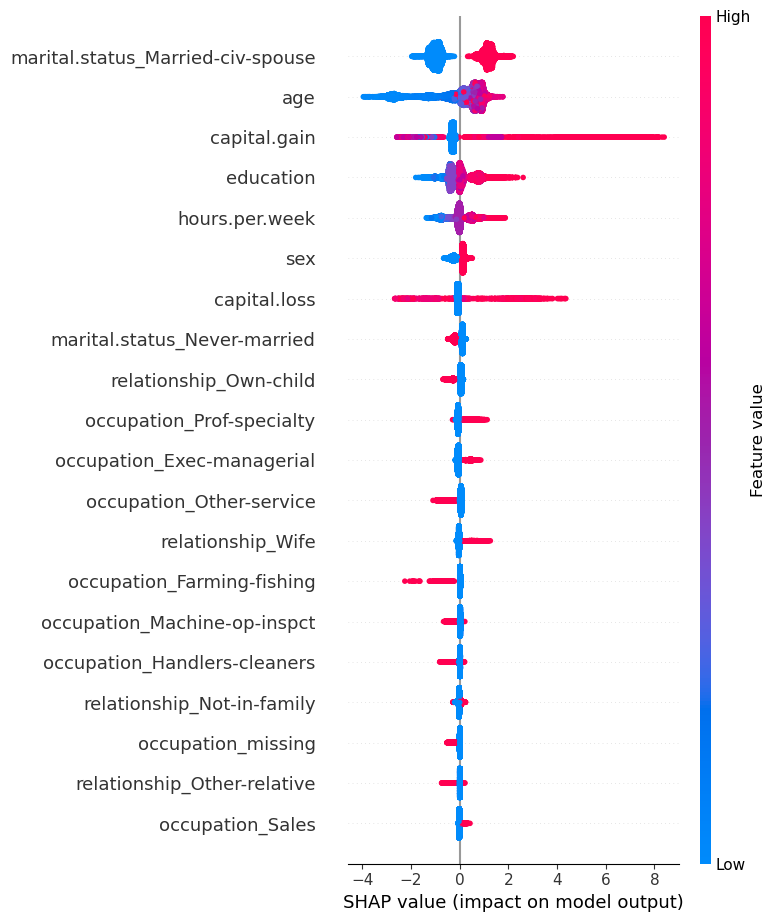
The plot shows the most important features for predicting the class. It also shows the direction of how it’s going to drive the prediction.
Presence of the marital status of Married-civ-spouse seems to have bigger SHAP values for class 1 and absence seems to have smaller SHAP values for class 1.
Higher levels of education seem to have bigger SHAP values for class 1 whereas smaller levels of education have smaller SHAP values.
shap.summary_plot(train_lgbm_shap_values[1], X_train_enc, plot_type="bar")
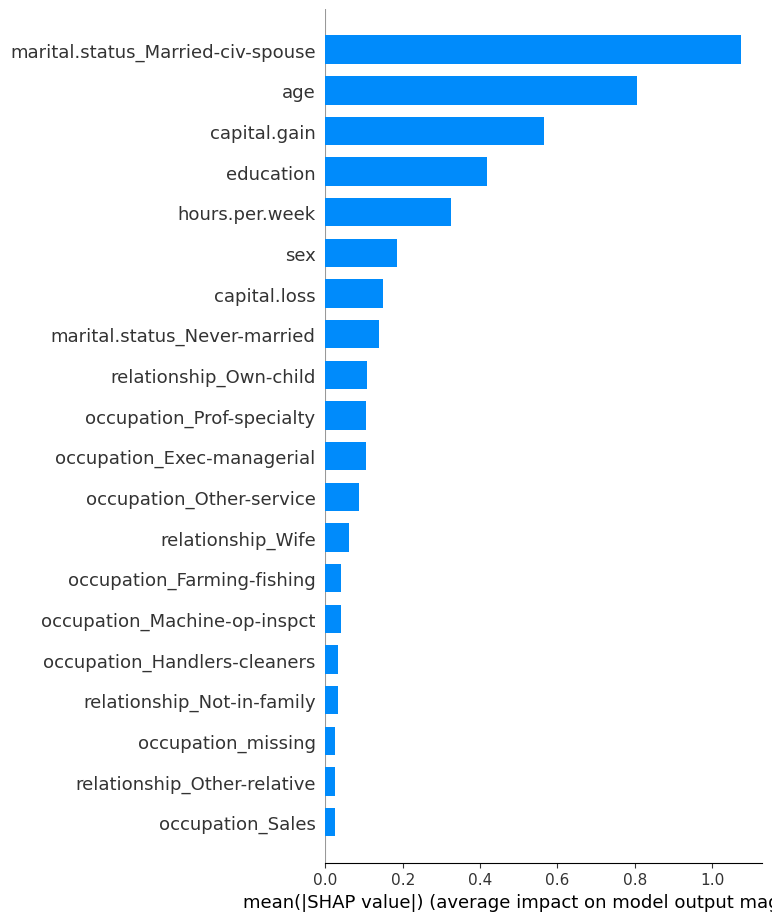
You can think of this as global feature importances.
Here, we explore SHAP’s TreeExplainer. It also provides explainer for different kinds of models.
TreeExplainer (supports XGBoost, CatBoost, LightGBM)
DeepExplainer (supports deep-learning models)
KernelExplainer (supports kernel-based models)
GradientExplainer (supports Keras and Tensorflow models)
Can also be used to explain text classification and image classification
Example: In the picture below, red pixels represent positive SHAP values that increase the probability of the class, while blue pixels represent negative SHAP values the reduce the probability of the class.
Other tools#
lime is another package.
If you’re not already impressed, keep in mind:
So far we’ve only used sklearn models.
Most sklearn models have some built-in measure of feature importances.
On many tasks we need to move beyond sklearn, e.g. LightGBM, deep learning.
These tools work on other models as well, which makes them extremely useful.
Why do we want this information?#
Possible reasons:
Identify features that are not useful and maybe remove them.
Get guidance on what new data to collect.
New features related to useful features -> better results.
Don’t bother collecting useless features -> save resources.
Help explain why the model is making certain predictions.
Debugging, if the model is behaving strangely.
Regulatory requirements.
Fairness / bias. See this.
Keep in mind this can be used on deployment predictions!
Here are some guidelines and important points to remember when you work on a prediction problem where you also want to understand which features are influencing the predictions.
Examine multicoliniarity in your dataset using methods such as VIF.
If you observe high correlations in your dataset, either get rid of redundant features or be mindful of these correlations during interpretation.
Be mindful that feature relevance is not clearly defined. Adding/removing features can change feature importance/unimportance. Also, feature importances do not give us causal relationships. See this optional section from Lecture 4.
Most of the models we use in ML are regularized models. With L2 regularization, the feature importances are distributed evenly among correlated features. With L1 regularization, one of the correlated features gets a high importance and the other gets a lower importance.
Don’t be overconfident. Always take feature importance values with a grain of salt.
What did we learn today?#
Why interpretation?
Interpretation in terms of feature importances
Interpretation beyond linear models with eli5
Making sense of SHAP plots
❓❓ Questions for you#
iClicker Exercise 8.1#
iClicker cloud join link: https://join.iclicker.com/C0P55
Select all the statements which are True.
(A) You train a random forest on a binary classification problem with two classes [neg, pos]. A value of 0.580 for feat1 given by
feature_importances_
attribute of your model means that increasing the value of feat1 will drive us towards positive class.(B) eli5 can be used to get feature importances for non
sklearn
models.(C) With SHAP you can only explain predictions on the training examples.
V’s answers: B
In what scenarios interpretability can be harmful?
Final comments#
If you’re curious about deployment, here are my notes from CPSC 330 on this topic.
Some key takeaways#
Some useful guidelines:
Do train-test split right away and only once
Don’t look at the test set until the end
Don’t call
fit
on test/validation dataUse pipelines
Use baselines
Do not be overconfident about your models. They do not tell us how the world works.
Difference between Statistics and Machine Learning#
There is no clear boundary.
But loosely
in statistics the emphasis is on inference (usually framed as hypothesis testing)
in machine learning the emphasis is on prediction and generalization. We are primarily interested in using our model on new unseen examples.
Recipe to approach a supervised learning problem with tabular data#
Understanding the problem#
Have a long conversation with the stakeholder(s) who will be using your pipeline.
Have a long conversation with the person(s) who collected the data.
Think about
what do you want to accomplish?
whether you really need to use machine learning for the problem or not
how do you want to frame the problem as a supervised machine learning problem? What do you want to predict?
how do you plan to measure your success?
is there a baseline?
is there any operating point?
what will it buy the stakeholders if you improve over their current baseline?
Think about the ethical implications - are you sure you want to do this project? If so, should ethics guide your approach?
Initial analysis, EDA, preprocessing#
Random train-test split with fixed random seed; do not touch the test data until Step 16.
Exploratory data analysis, outlier detection.
Choose a scoring metric -> higher values should make you & your stakeholders happier.
Feature engineering –> Create new features which might be useful to solve the prediction problem. This is typically a time-consuming process. Also, sometimes you get an idea of good features only after looking at your model results. So it’s an iterative process.
Fit a baseline model, e.g.
DummyClassifier
orDummyRegressor
.Create a preprocessing pipeline.
(Optional) Incorporate feature selection.
Model building#
Try a linear model, e.g.
LogisticRegression
for classification orRidge
; tune hyperparameters with CV.Try other sensible model(s), e.g. LightGBM; tune hyperparameters with CV.
For each model, look at sub-scores from the folds of cross-validation to get a sense of “error bars” on the scores.
Pick a few reasonable models. Best CV score is a reasonable metric, though you may choose to favour simpler models.
Carry out hyperparameter optimization for these models, paying attention to whether you are not susceptible to optimization bias.
Try averaging and stacking to examine whether you improve the results over your best-performing models.
Model transparency and interpretation#
Look at feature importances.
(optional) Perform some more diagnostics like confusion matrix for classification, or “predicted vs. true” scatterplots for regression.
(optional) Try to calibrate the uncertainty/confidence outputted by your model.
Test set evaluation.
Model transparency. Take a random sample from the test data and try to explain your predictions using SHAP plots.
Question everything again: validity of results, bias/fairness of trained model, etc.
Concisely summarize your results and discuss them with stakeholders.
(optional) Retrain on all your data.
Deployment & integration.
PS: the order of steps is approximate, and some steps may need to be repeated during prototyping, experimentation, and as needed over time.
Coming up#
We haven’t talked about deep learning and working with images yet. It’s coming up in block 5 (DSCI 572).
Supervised learning is quite successful but many well-known people in the field believe that it’s fundamentally limited, as it doesn’t seem to be how humans learn. We’ll learn about unsupervised learning in block 5 (DSCI 563).
Forecasting and time series is another important area in machine learning. You’ll be learning about it in block 5 (DSCI 574)
Finally, we’ll talk about sequential models for text data in block 6 (DSCI 575)
Farewell#
That’s all, folks. We made it! Good luck with the quiz next week. Happy holidays and I’ll see you in block 5!
Time for course evaluations#
We are happy to hear your thoughts on this course. Here is the link: https://canvas.ubc.ca/courses/102041/external_tools/4732