PageRank as a Markov model#
PageRank: intuition#
Important webpages are linked from other important webpages.
Don’t just look at the number of links coming to a webpage but consider who the links are coming from
PageRank: scoring#
Imagine a browser doing a random walk
At time t=0, start at a random webpage.
At time t=1, follow a random link on the current page.
At time t=2, follow a random link on the current page.
Intuition
In the “steady state” each page has a long-term visit rate, which is the page’s score (rank).
PageRank: teleporting#
Obvious problem with the random walk:
Pages with no in-links have a rank of 0.
Algorithm can get “stuck” in part of the graph, as the web is full of dead-ends.
PageRank: teleporting#
At a dead end, jump to a random web page.
At a non-dead end, we still jump to a random web page with probability \(\alpha\).
With the remaining probability \((1-\alpha)\) go out on a random link.
With teleporting, we cannot get stuck locally.
Questions#
PageRank as a Markov chain#
A state is a web page
Transition probabilities represent probabilities of moving from one page to another
We derive these from the adjacency matrix of the web graph
Adjacency matrix \(M\) is a \(n \times n\) matrix, if \(n\) is the number of states (web pages)
\(M_{ij} = 1\) if there is a hyperlink from page \(i\) to page \(j\).
PageRank: deriving transition matrix#
If a state has no out-links, the random surfer teleports:
the transition probability to each state from this state is \(1/n\), if \(n\) is the number of states
If a node has K > 0 outgoing links:
with probability \(0 \leq \alpha \leq 1\) the surfer teleports to a random node
probability is \(\alpha/n\)
with probability \((1-\alpha)\) the surfer takes a normal random walk
probability is \((1-\alpha)/K\)
PageRank: deriving transition matrix#
If a row has all 0’s, replace each element by \(1/n\)
Else
Normalize: divide each 1 by the number of 1’s in the row
Multiply the resulting matrix by \((1-\alpha)\)
Add \(\alpha/n\) to every element in the resulting matrix to create the transition matrix \(A\).
PageRank: deriving transition matrix (example)#
Adjacency matrix: $ M =
, \alpha = 0.0$
Transition matrix: $ A_{\alpha=0} =
PageRank: deriving transition matrix (example)#
$ M =
, n =3, \alpha = 0.5$
Normalize: divide each 1 by the number of 1’s in the row:
$
PageRank: deriving transition matrix (example)#
$ M =
, n =3, \alpha = 0.5$
Normalized
Multiply the resulting matrix by \((1-\alpha)\): $ (1-0.5)
$ =
Add \(\alpha/n\) to every element in the resulting matrix to create the transition matrix: $ A_{\alpha = 0.5} =
Questions#
Calculate page rank#
We have a transition matrix: $A_{\alpha = 0.5} =
We want to find the stationary distribution
Is it irreducible?
Is it aperiodic?
Calculate page rank: power iteration method#
Start with a random initial probability distribution \(\pi\)
Multiply \(\pi\) by powers of the transition matrix \(A\) until the product looks stable
After one step, we are at \(\pi A\)
After two steps, we are at \(\pi A^2\)
After three steps, we are at \(\pi A^3\)
Eventually (for a large \(k\)), \(\pi A^k = \pi\)
How to do this in Python?#
Use networkx package google_matrix function. Warning: their definition of 𝛼 is opposite from ours.
import networkx as nx
G=nx.Graph()
G.add_node('A')
G.add_node('B')
G.add_node('C')
G.add_edge('A','B')
G.add_edge('B','A')
G.add_edge('B','C')
G.add_edge('C','B')
nx.draw(G, with_labels=True)
plt.show()
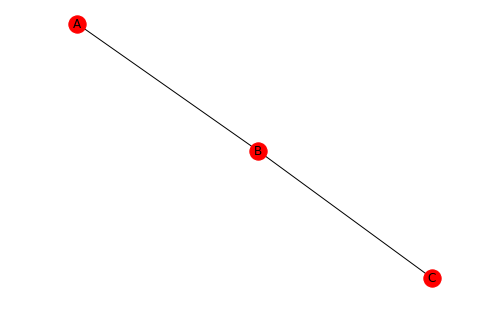
# Transition matrix for alpha = 0.0
print(nx.google_matrix(G, nodelist=sorted(G.nodes()), alpha=1.0 - 0.0))
[[0. 1. 0. ]
[0.5 0. 0.5]
[0. 1. 0. ]]
# Transition matrix for alpha = 0.5
A = nx.google_matrix(G, nodelist=sorted(G.nodes()), alpha = 1.0 - 0.5)
print(A)
[[0.16666667 0.66666667 0.16666667]
[0.41666667 0.16666667 0.41666667]
[0.16666667 0.66666667 0.16666667]]
def print_pi_over_time(s0, T, steps=10):
prev = s0
print('Step 0', prev)
for i in range(steps):
current = prev@T
print('Step ', i+1, current)
prev = current
# Random initialization of pi
pi = [.4, 0.2, 0.2]
# Power iteration method
print_pi_over_time(pi, A, steps=40)
Step 0 [0.4, 0.2, 0.2]
Step 1 [[0.18333333 0.43333333 0.18333333]]
Step 2 [[0.24166667 0.31666667 0.24166667]]
Step 3 [[0.2125 0.375 0.2125]]
Step 4 [[0.22708333 0.34583333 0.22708333]]
Step 5 [[0.21979167 0.36041667 0.21979167]]
Step 6 [[0.2234375 0.353125 0.2234375]]
Step 7 [[0.22161458 0.35677083 0.22161458]]
Step 8 [[0.22252604 0.35494792 0.22252604]]
Step 9 [[0.22207031 0.35585937 0.22207031]]
Step 10 [[0.22229818 0.35540365 0.22229818]]
Step 11 [[0.22218424 0.35563151 0.22218424]]
Step 12 [[0.22224121 0.35551758 0.22224121]]
Step 13 [[0.22221273 0.35557454 0.22221273]]
Step 14 [[0.22222697 0.35554606 0.22222697]]
Step 15 [[0.22221985 0.3555603 0.22221985]]
Step 16 [[0.22222341 0.35555318 0.22222341]]
Step 17 [[0.22222163 0.35555674 0.22222163]]
Step 18 [[0.22222252 0.35555496 0.22222252]]
Step 19 [[0.22222207 0.35555585 0.22222207]]
Step 20 [[0.2222223 0.35555541 0.2222223 ]]
Step 21 [[0.22222219 0.35555563 0.22222219]]
Step 22 [[0.22222224 0.35555552 0.22222224]]
Step 23 [[0.22222221 0.35555557 0.22222221]]
Step 24 [[0.22222223 0.35555555 0.22222223]]
Step 25 [[0.22222222 0.35555556 0.22222222]]
Step 26 [[0.22222222 0.35555555 0.22222222]]
Step 27 [[0.22222222 0.35555556 0.22222222]]
Step 28 [[0.22222222 0.35555555 0.22222222]]
Step 29 [[0.22222222 0.35555556 0.22222222]]
Step 30 [[0.22222222 0.35555556 0.22222222]]
Step 31 [[0.22222222 0.35555556 0.22222222]]
Step 32 [[0.22222222 0.35555556 0.22222222]]
Step 33 [[0.22222222 0.35555556 0.22222222]]
Step 34 [[0.22222222 0.35555556 0.22222222]]
Step 35 [[0.22222222 0.35555556 0.22222222]]
Step 36 [[0.22222222 0.35555556 0.22222222]]
Step 37 [[0.22222222 0.35555556 0.22222222]]
Step 38 [[0.22222222 0.35555556 0.22222222]]
Step 39 [[0.22222222 0.35555556 0.22222222]]
Step 40 [[0.22222222 0.35555556 0.22222222]]
Modern ranking methods are more advanced:#
Guarding against methods that exploit algorithm.
Removing offensive/illegal content.
Supervised and personalized ranking methods.
Take into account that you often only care about top rankings.
Also work on diversity of rankings:
E.g., divide objects into sub-topics and do weighted “covering” of topics.
Persistence/freshness as in recommender systems (news articles).